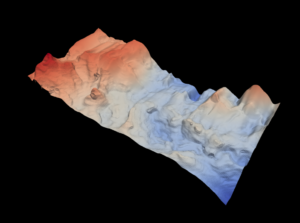
Overview: We have been using machine learning (ML) for over a decade as a means of accelerating computationally expensive simulations, exploring materials design spaces, and developing surrogates for highly nonlinear relationships. Our training data often come from high-fidelity numerical simulations, including molecular dynamics simulations and crystal-plasticity simulations. We have been some of the first to apply machine learning in the field of fracture mechanics. In the MMM Lab, we continue to explore the cutting edge of ML and AI while harnessing our domain expertise in materials modeling and fracture mechanics to solve problems in materials and structural engineering that were previously unsolvable.
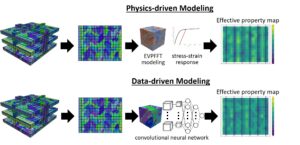
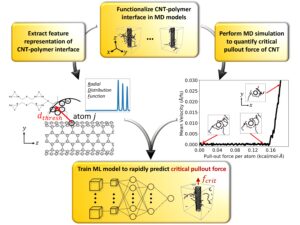
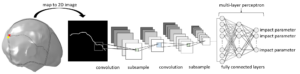
Select publications (MMM Lab members are underscored):
- K.J. DeMille, A.D. Spear, Convolutional Neural Networks for Expediting the Determination of Minimum Volume Requirements for Studies of Microstructurally Small Cracks, Part II: Model Interpretation, Computational Materials Science, Vol. 227, pp. 112261, 2023. https://doi.org/10.1016/j.commatsci.2023.112261
- K.J. DeMille, A.D. Spear. Convolutional Neural Networks for Expediting the Determination of Minimum Volume Requirements for Studies of Microstructurally Small Cracks. Part I: Model Implementation and Predictions, Computational Materials Science, Vol. 207, pp. 111290, 2022. https://doi.org/10.1016/j.commatsci.2022.111290
- A. Rahman, P. Deshpande, M. Radue, G. Odegard, S. Gowtham, S. Ghosh, A.D. Spear. A Machine Learning Framework for Predicting the Shear Strength of Carbon Nanotube-Polymer Interfaces Based on Molecular Dynamics Simulation Data, Composites Science and Technology, Vol. 207, pp. 108627, 2021. https://doi.org/10.1016/j.compscitech.2020.108627
- C. Herriott, A.D. Spear. Predicting Microstructure-dependent Mechanical Properties in Additively Manufactured Metals with Machine- and Deep-learning Methods, Computational Materials Science, Vol. 175, pp. 109599, 2020. doi.org/10.1016/j.commatsci.2020.109599
- N. Shirolkar, P. Patwardhan, A. Rahman, A.D. Spear, S. Kumar. Investigating the Efficacy of Machine Learning Tools in Modeling the Continuous Stabilization and Carbonization Process and Predicting Carbon Fiber Properties, Carbon, Vol. 174, pp. 605-616, 2021. https://doi.org/10.1016/j.carbon.2020.12.044
- K. Pierson, A. Rahman, A.D. Spear. Predicting Microstructure-sensitive Fatigue-crack Path in 3D Using a Machine Learning Framework, JOM, Vol. 71(8), pp. 2680-2694, 2019. doi.org/10.1007/s11837-019-03572-y
- A.D. Spear, A.R. Priest, M.G. Veilleux, J.D. Hochhalter, A.R. Ingraffea. Surrogate Modeling of High-fidelity Fracture Simulations for Real-time Residual Strength Predictions, AIAA Journal, Vol. 49, No. 12, pp. 2770-2782, 2011. doi:10.2514/1.55295