Research Pillars
Computational Fracture Mechanics
Overview: We develop and augment a variety of numerical tools to simulate complex and realistic fracture behavior in 3D. Our tools have been used to simulate crack growth in 3D polycrystalline materials, including additively manufactured metals and other aerospace materials, as well as biological materials, including human bone.
Select publications: IntJPlasticity2023, CompMaterialsScience2021, BiomechanModelingMechanobiology2020, IntJFracture2020, IntJFracture2019, EngFractureMech2019
Machine Learning and Materials Informatics
Overview: We have been using machine learning (ML) for nearly a decade as a means of accelerating computationally expensive simulations, exploring materials design spaces, and developing surrogates for highly nonlinear relationships. Our training data often come from high-fidelity numerical simulations, including molecular dynamics simulations and crystal-plasticity simulations. We have been some of the first to apply machine learning in the field of fracture mechanics.
Select publications:
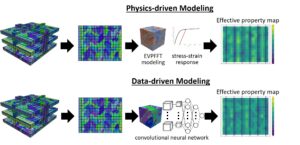
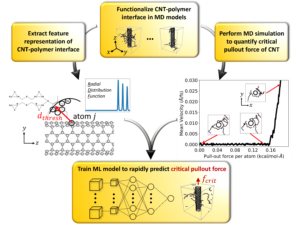
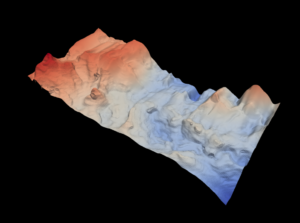
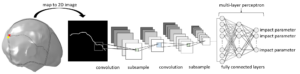
Additive Manufacturing (3D Printing)
Overview: We study the deformation and failure mechanisms in additively manufactured metals, bringing to bear the power of our numerical tools and experimental observations. Our group has participated in (and won) several international modeling challenges involving 3D-printed metals. Prof. Spear led the acquisition of the University of Utah's first laser powder bed fusion machine, which we leverage to support our research.
Select publications:
Cellular Materials
blah blah